SEARCH
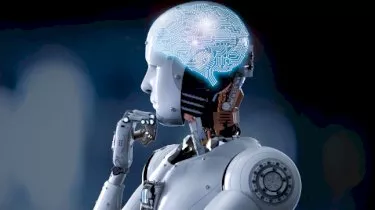
FASEA code to encourage adviser takeup of AI
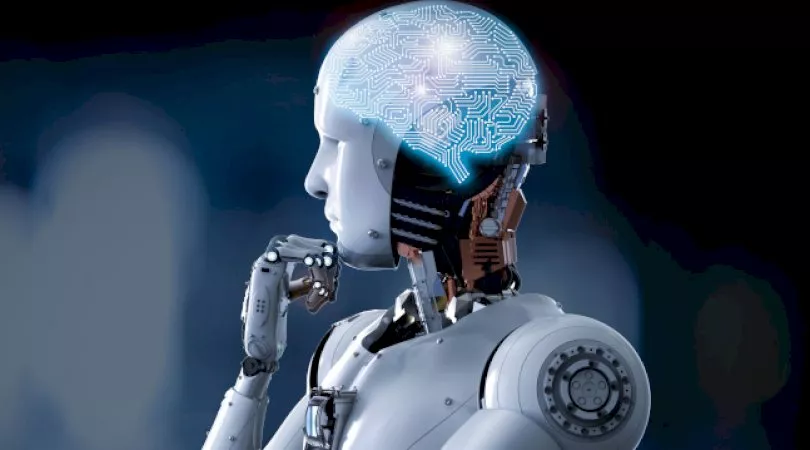
FASEA code to encourage adviser takeup of AI
A financial education provider says the new FASEA Code of Ethics provides a good opportunity for advisers to leverage the full potential of artificial intelligence and machine learning technology.
According to Kaplan Professional chief executive Brian Knight, with FASEA's Code of Ethics coming to effect on 1 January 2020, now is a suitable time for the industry to look to maximise the assistance of technology.
"Over the next 12 months, the industry needs to see this as an opportunity to be much clearer and crisper in its documentation and procedures," he said.
"It needs to be down to the level where the industry can utilise these technologies to assist those on the front line, while training the next generation and retraining existing advisers on their exact obligations."
Regtech firm Red Marker said there needed to be a mindset change within the advice industry on how AI and machine learning tools can best assist the industry.
"It is important both dealer groups and vendors progress with realistic expectations, particularly around the 'pre-work' that needs to be done to ensure financial advice can become an ideal candidate for automated solutions," said Red Marker CEO Matt Symons.
"If the financial services industry wants to increase the likelihood that effective statement of advice (SoA) review solutions emerge at a faster rate, then we need to come together and collaborate … working together is going to be key to developing highly-reliable, automated review solutions."
However, both Kaplan Professional and Red Marker noted that, before the industry can benefit from advice review technologies built using AI and machine learning, existing pre-conditions needed to be in place, including:
- Expectations need to be managed - automated solutions will not be available 'off-the-shelf' to replace or meaningfully reduce the amount of supervision required.
- Although significant training data exists, there are limitations, including:
a. Many files require optical character recognition, and the quality of data extraction is challenging for natural language processing and machine learning techniques.
b. Regulations have evolved in recent times and what once might have been acceptable may not be any more, limiting the 'training' value of this data record.
- The industry seems to be diverging, rather than converging on standard approaches to SoA construction, automatic programming language and product comparison logic.
Subscribe to our Newsletter
We Translate Complicated Financial Jargon Into Easy-To-Understand Information For Australians
Your email address will be shared with nestegg and subject to our Privacy Policy
latest articles
OUR PLATFORMS AND BRANDS
- Accountants Daily
- Accounting Times
- Adviser Innovation
- Australian Aviation
- Broker Daily
- Cyber Daily
- Defence Connect
- Fintech Business
- HR Leader
- Independent Financial Adviser
- Investment Centre
- Investor Daily
- Lawyers Weekly
- Money Management
- Nestegg
- Property Buzz
- Real Estate Business
- Smart Property Investment
- SMSF Adviser
- Space Connect
- Super Review
- The Adviser
- Wellness Daily
- World of Aviation
EVENTS AND SUMMITS
- Accountants Daily 30 Under 30 Awards
- ACE25
- Adviser Innovation Summit
- Australian Accounting Awards
- Australian Aviation Awards
- Australian Broking Awards
- Australian Defence Industry Awards
- Australian Law Awards
- Australian Space Awards
- Australian Space Summit
- Better Business Summit & Awards
- Broker Daily Business Awards
- Corporate Counsel Summit & Awards
- Cyber Security Summit & Awards
- Defence Connect Budget Lunch
- Defence Connect DSR Summit
- Fund Manager of the Year Awards
- ifa Excellence Awards
- ifa Future Forum
- Investor Daily ESG Summit
- Lawyers Weekly 30 Under 30 Awards
- Lawyers Weekly Women in Law Forum
- New Broker Academy
- Partner of the Year Awards
- REB Awards
- Reinnovate
- SME Broker Bootcamp
- SMSF Adviser Technical Day
- Super Fund of the Year Awards
- Women in Finance Awards
- Women in Law Awards
- Accountants Daily Podcast Network
- Australian Aviation Podcast Network
- Broker Daily Podcast Network
- Defence Connect Podcast Network
- HR Leader Podcast Network
- REB Podcast Network
- Relative Return
- Space Connect Podcast
- The Adviser Podcast Network
- The ifa Show
- The Lawyers Weekly Show
- The Smart Property Investment Show
PODCASTS
LEARNING AND EDUCATION
MOMENTUM MARKETS NETWORK
LINKS
STAY CONNECTED
Subscribe to the Adviser Innovation eNewsletter.
OUR PLATFORMS AND BRANDS
- Accountants Daily
- Accounting Times
- Adviser Innovation
- Australian Aviation
- Broker Daily
- Cyber Daily
- Defence Connect
- Fintech Business
- HR Leader
- Independent Financial Adviser
- Investment Centre
- Investor Daily
- Lawyers Weekly
- Money Management
- Nestegg
- Property Buzz
- Real Estate Business
- Smart Property Investment
- SMSF Adviser
- Space Connect
- Super Review
- The Adviser
- Wellness Daily
- World of Aviation
EVENTS AND SUMMITS
- Accountants Daily 30 Under 30 Awards
- ACE25
- Adviser Innovation Summit
- Australian Accounting Awards
- Australian Aviation Awards
- Australian Broking Awards
- Australian Defence Industry Awards
- Australian Law Awards
- Australian Space Awards
- Australian Space Summit
- Better Business Summit & Awards
- Broker Daily Business Awards
- Corporate Counsel Summit & Awards
- Cyber Security Summit & Awards
- Defence Connect Budget Lunch
- Defence Connect DSR Summit
- Fund Manager of the Year Awards
- ifa Excellence Awards
- ifa Future Forum
- Investor Daily ESG Summit
- Lawyers Weekly 30 Under 30 Awards
- Lawyers Weekly Women in Law Forum
- New Broker Academy
- Partner of the Year Awards
- REB Awards
- Reinnovate
- SME Broker Bootcamp
- SMSF Adviser Technical Day
- Super Fund of the Year Awards
- Women in Finance Awards
- Women in Law Awards
PODCASTS
- Accountants Daily Podcast Network
- Australian Aviation Podcast Network
- Broker Daily Podcast Network
- Defence Connect Podcast Network
- HR Leader Podcast Network
- REB Podcast Network
- Relative Return
- Space Connect Podcast
- The Adviser Podcast Network
- The ifa Show
- The Lawyers Weekly Show
- The Smart Property Investment Show